Field service work has a variety of constraints and special characteristics that may influence how the flow of field service work is managed using kanban. What is different about field service and what should we take into account when using kanban to manage field service work?
Many of the issues that I will describe may possibly be addressed by changing the value stream and by simply removing the need to perform field service (for example, by using remote controls). However advisable and useful that approach might be, I assume that as long as there will be physical equipment at a customer’s premises, there will always be a need for field service. Consequently, these remarks remain valid for what field service acts persist. If remote service is instituted, then that service is out of the scope of this discussion.
In this article, I will concentrate on the following themes:
- The wastes of waiting and transportation, both on the provider side and the customer side
- Planning the start of interventions and selecting the next kanban card
- Card board mobility
- Field service agent assignment and resource liquidity
What is special about field service?
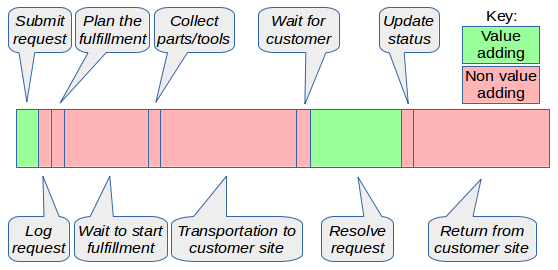
We may analyze the specifics of field service from a lean, value-adding/non-value-adding, perspective (see Fig. 1). Field service, by definition, includes a significant amount of waste in the form of transportation. Each field service support request requires the displacement of the agent and possibly his or her tools to a remote site. In addition, field service is often only performed during business hours. The waiting time until the start of service may be exacerbated by the need to wait until a future business day. Additional waiting may be incurred if the demand for field service momentarily outstrips the supply of agents.

Traditional field service management tools are designed to minimize the overall waste due to transportation. Such optimization is a classic application of solutions to the traveling salesperson problem. To give a simple example, suppose there are three calls requiring field service and a single agent available to perform the service. All things being equal, the service visits might be scheduled according to a first-in, first-out queue (see Fig. 2). But suppose the second customer in a queue is 20 kms distant from the first customer, whereas the third customer in the queue is a next door neighbor of the first one. The visit to the third customer might be scheduled before the third to minimize the waste of traveling by the agent (see Fig. 3).

There are limits, however, to the benefits that route optimization, by itself, can procure. Let’s look at a somewhat oversimplified example (see Fig. 4). Suppose an agent visits an average of five sites per day, that is, an average of about 100 minutes per site, given an 8.5 hours workday. Suppose, further, that 60 of those 100 minutes are spent in issue resolution and nearly 40 minutes in transportation. If we wanted to increase the average resolved visits per day to six that would be an average of about 85 minutes per site. There is no point to increasing a fraction of a resolved visit, as this would imply return visits and the associated wastes of transportation and waiting.
To achieve this route optimization we would have to reduce the average transportation time per site by more than 15 minutes, an improvement of at least 37%. I can’t say that such improvements are impossible, but I do think they are highly unlikely based on route optimization alone. If we look at some examples of heuristic solutions to the traveling salesperson problem, we see that compared to a naïve routing algorithm, such as “go to the closest site next”, it might be hard to get more than a 15% improvement. Thus, route optimization might help to absorb risks in transportation, or allow for extra time to resolve issues, or even provide additional spare time to the agents to support improvements. Such optimization is on the same order of magnitude as the improvements that kanban can bring to the flow of work. However, route optimization would not increase the number of visits per day (in our example) unless:
- Overtime solution: the agents were also to work overtime (but in a lean approach to managing work, it is precisely such employee overburdening, or muri, that we seek to avoid); or
- Two visit solution: we accept that many of the service calls at the end of the day will not resolve the issue and will require a return visit (in which case the transportation and waiting wastes are doubled and we would still have to work overtime).
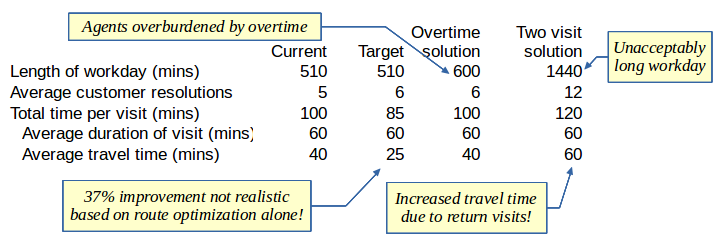
One easily sees that in cases where there are thousands of customers and dozens of agents, the optimization of scheduling can become extremely complex. This complexity is due to the non-deterministic nature of the traveling salesperson problem and all the standard queuing theory factors, such as the number of agents, the desired service levels, the mean duration of tasks, and so forth, influence the scheduling and routing of the service agents.
Service levels and customer expectations
The idea of service levels is an important factor providing the customer’s presumed point of view of what makes for good service. The customer is interested in three factors:
- the start time of the intervention
- the difference between the expected start time and the actual start time
- the finish time of successful field service
In many cases, excepting the most standardized of services, there can hardly be a planned finish time until the agent has seen the issue first hand. Thus, the finish time will not usually be framed by promises from the provider.1
From the objective perspective of the final value to the customer of the service act, the start time of the intervention is irrelevant. The customer should be concerned only with the overall loss of value due to the need for support. In most cases, this depends on when the issue is resolved, not on when the diagnosis of the issue starts. However, from long years of experience, the customer has come to expect a relationship between the start time of diagnosis and the end time of resolution. The customer distinguishes between the perception that the provider is “doing something” and the perception that the provider is “doing nothing” or, at best, “busy working for someone else”. Thus, customers are similar to traditional managers who are more concerned with keeping their teams busy than with improving the lead times for work, believing that the latter depends on the former. Kanban has shown that this assumption is not only false, but typically exactly the opposite of what one might suppose.
There is, however, an objective reason for planning a start time for field service. Between the time that a customer requests support and the time that support starts there is likely to be a certain amount of waiting, a second form of waste. That waiting is of significant psychological value, too, if the customer fidgets for long hours should the agent arrive long after planned. Thus, if the planned arrival time is “this afternoon” (that is, between 14:00 and 18:00) and the actual arrival time is 17:00, the customer may have wasted up to three hours of waiting time. The more precise the planned start time and the more accurate the actual start time compared to the planned start time, the less the potential for waste on the customer’s side.
Finally, the actual resolution time, or the overall lead time for the work, is an important service level for the customer. Unlike services performed remotely, field service is particularly subject to additional delays should the issue not be resolved by the agent during the first visit. Should that agent have to return a second time, lacking a part or some type of information; should the agent require the help of yet another agent to resolve the issue; in these cases the lead time is subject to the wastes of transportation and waiting already described for the first visit by the agent.
Drawing the next card
How do these special factors influence the management of the flow of work using kanban? The first issue is with planning the start of the intervention. In a strict application of kanban, the commitment to do work should be delayed as late as possible. That commitment would be the planning for when the agent first arrives at the customer’s site. By delaying the planning until an agent has completed the previous support visit, there are several advantages. First, there is relatively little uncertainty regarding when the intervention could start. When planning is made during the initial request for support, there is often a great deal of uncertainty regarding the duration of higher priority interventions. Since field service agents are unwilling to commit to times they will not be able to respect, commitment is made only to vague lapses of time: “in the afternoon”, etc. If, instead, the planning is delayed until the agent knows he or she will be available, the principal factor of risk is delay in the transportation to the customer’s site.
The difficulty with this purist approach is that the customer is not necessarily going to be available, given the relatively short notice, when the agent plans to be available. This is especially true when the intervention is at the customer’s home, but may also be true for the workplace. There are two approaches to managing this issue:
- Pre- or tentative commitment to a start date
- Using customer availability as a factor in prioritization
Let’s look at an example, as shown in Fig. 5. An agent starts the work day at 8:00 (1), performing support job 1. Around 8:45 (2) a call comes in for a different job, one that this agent is likely to perform. At the time of that call, the breadth of the estimate for when the agent could start the second support job is quite wide. Suppose there is a 90% chance that it can start some time between 13:00 to 17:00. At around 10:00 (3), the agent has advanced sufficiently in support job 1 to be able to better estimate when it will end and, consequently, when support job 2 could start. At 10:00, suppose there is a 90% probability that it can start between 14:00 and 15:00. At around 13:00 (4), the first support job is completed and the only uncertainty regarding the start of the second support job concerns the time of transportation to the customer’s site. The actual arrival time and the start of the second support job is 14:45 (5).

Thus, we see that the service provider can make successive estimates for the start of the support job, based on the state of the current work, the information provided by the customer and the historical record for work on the current support job. When the customer first calls, a pre-commitment by the agent may be made, with a broad estimate of availability. Later, when the probable end of the current job is better known, a full commitment could be made, based on the estimated transportation time and the estimated end of the current job.
From a kanban perspective, the agent would need to do three things:
- Note the pre-commitment on the kanban card for the second support job. That card would either remain in the Ready column or, a “Pre-commitment” column could be created, if the latter option simplified handling.
- When the first support job is completed, the corresponding kanban card would be moved to the Done column (or perhaps to some other column, such as “Ready for invoicing”).
- The card for the second support job, on which the pre-commitment was noted, is moved to the “Support in progress” column, moving it either from the Ready column or the Pre-commitment column, depending on the solution used.
But suppose when the pre-commitment passes to a more formal commitment, the customer says that he or she cannot be available at that time, or is not even available to confirm the start time. What should happen?
The risk is that the agent will appear at the second support site but then be obliged to wait an indefinite amount of time. This form of waste is would be compounded by the waste of the transportation time, should the job have to be abandoned. To mitigate these risks, the agent can consider the pre-commitment to be nullified, move the kanban card back into the Ready column, and then choose another job based on the job selection policies in place (although sometimes the best option is to have to wait for the customer).
You might object that using pre-commitments increases the co-ordination overhead and the risk of error. The coming availability of robotic assistants that can automate the contact with the customer will relieve the agent of that overhead.
Mobile card boards
Each field service agent should have remote and mobile access to the team’s card board. There are three unsatisfactory alternatives to such access:
We finally called the chimney sweep, only to find that she had not planned to visit us today. Although we had both written down the appointment during her previous visit, the note that she had written on the back of an envelope never made it to the official list of work orders.
- Having to return to a central office after each job is completed (huge additional waste in transportation and in waiting)
- Calling in to a central office where the card board is located and have another person update the card board and communicate status information (waste of handing off tasks to another person and forcing that person to be available). However, this issue might be mitigated by the use of robotic call assistants
- Not updating the card board until the agent returns to a central office, often at the end of the working day (low agility, difficulty in handling expedited work)
We can easily imagine mobile access to a virtual card board via a telephone or tablet. That device may have a docking station in the agent’s vehicle (see Fig. 6), ensuring its charging and easy visibility during transportation. Autonomously driven vehicles may reduce the danger of consulting the card board while on the road.
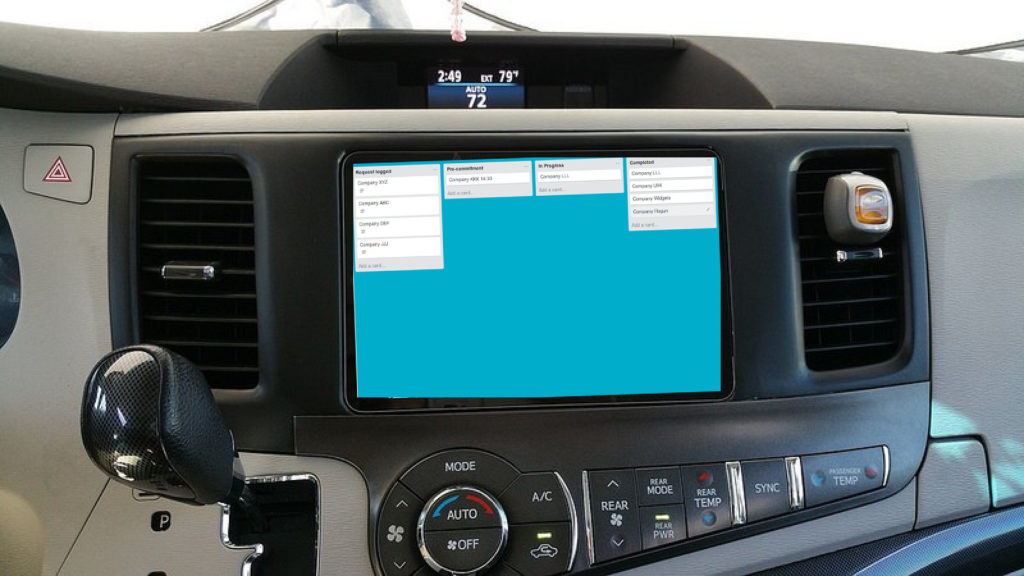
In some cases, typically when field service is delivered at an office with a permanent presence that does not require advance notification of the arrival hour, a mobile card board allows the agent to use kanban in a standard way. That is to say, upon completion of a visit, the agent updates the status of the completed work order and then selects the next work order from the upstream Ready column.
While this approach might be optimal from the perspective of smoothing the flow of work, it is perhaps not acceptable when the customer expects the agent’s arrival at a certain hour. In this case, the agent needs to see on the card board the expected arrival times, so that the appropriate next work order may be selected and moved to the In Progress column. Such a class of service resembles the Fixed Date class, but depends on the expected start date rather than the required end date.
Assigning an agent to a work order
How and when is it decided that a given agent will handle a given work order? The later that assignment is made, the better the flow of work. In a classic kanban approach the assignment of the agent occurs when the agent selects the next work order to be performed. However, in field service other forces may mitigate against assignment as late as possible:
- The constraint of fixed routes
- The constraint of stable relationships between agents and customers
- The constraint of special knowledge required for a work order
- The constraint of limited access to tools and spare parts
Our government has, in its wisdom, decided that each region shall have only one chimney sweep company, a sort of utility monopoly. Periodic control of chimneys is mandatory, so the success of the chimney sweep is largely assured. In our case, we always have the same chimney sweep visit us, although each time she comes with a different apprentice.
Contrast this with the local volunteer fire department, which is also a monopoly. But the firefighters periodically visit each building in the commune to learn where the sources of water and the external fuse boxes are located. As a result, any group of firefighters will already be familiar with our house, should it catch fire. Precious time will be saved.
All these constraints serve to reduce resource liquidity and thereby can cause blockages in flow and lengthen lead times.
Fixed routes
Each agent might have a pre-assigned route or region for which she or he is responsible. In such a case, the assignment of agents to work orders is largely automatic, based on the location of the customer. Changes to such assignments are exceptions to the rule. They might be required when a agent is absent from work, when a customer is not on any existing route or when conflicting expedited work orders require one agent to venture into another agent’s region.
If we analyze this issue using the tools of graph theory, a region would be composed of a series of nodes, connected by routes, or edges. Each edge would have a weight, representing the “cost” of traveling from node to node. That “cost” could be in terms of distance, or travel time, or the monetary value of the displacement, etc. When the cost is measured in travel time, a region with heavily weighted edges would have a lot of transportation waste. Thus, it would make sense to define separate routes in the regions as a way to limit that waste.
However, the other factor that must be taken into account is the predictability of the distribution of work orders within a team’s region. Unless the support is part of planned maintenance or upgrades, the work orders are likely to be difficult to predict in advance, except as averages over a period of time. But I don’t want to manage on the basis of averages. I don’t want nearly all field service visits to be an average amount of time late (see Fig. 7). What I do want is for nearly all visits to be on time, other than the few, inevitable, delays that bring the average up (see Fig. 8).


Regular tuning and adjustment of routes represents a rich occasion for improving the on-time arrival of agents at customer sites and reduction in the waste of waiting.
Stable relationships
It may happen that a particular agent has an excellent relationship with a particular customer, leading to a high level of customer satisfaction. While this good relationship might be highly satisfactory, it also reduces resource liquidity and thereby reduces the overall flow of field service work.
There are two approaches to handling this situation. On the one hand, you can treat the relationship as a “best practice”, trying to understand how to reproduce it among the other agents and customers. On the other hand, you might prefer high liquidity to stability in relationships.
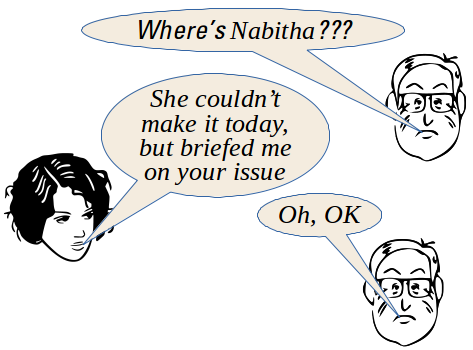
Special knowledge
The classic example of a bottleneck in the flow of work is the situation where only one person in a team has the knowledge or experience required to fulfill a customer request. Such issues are compounded in field service because of the risk of having to repeat the waste of transportation should a second, more knowledgeable, agent have to visit the customer to resolve the issue.
The question of how to address the problem of special knowledge is not specifically related to the management of the flow of work or kanban. The exception would be in case the agents work in pairs, as opposed to individually. Whatever advantages working in pairs might have,2 it once again faces the double whammy of having to transport two resources rather than one.
Other than working with an apprentice, doing field service in pairs remains very rare when one agent should be capable of performing the work. Consequently, this natural method for sharing knowledge and for mitigating the constraint of special knowledge is not often available to organizations. There are other methods for sharing special knowledge—technical, procedural and social. The applicability of these methods depends on the culture of the organization and its maturity levels. No single method is likely to be the most suitable among all organizations for improving overall knowledge sharing.3

Limited tools and spare parts
Imagine that a customer calls for service. Upon arriving at the site, the agent discovers that a very expensive diagnostic tool would be required to determine the cause of the problem. Owing to the cost of that tool, it is not feasible to provide one to each agent. Or perhaps there is limited space in the vehicle used to visit the customer sites, making it necessary to carry only the most commonly used tools and spare parts.
In such cases, it might be impossible for the agent to resolve the support call during the first visit. What is more, a second visit might have to scheduled only after getting a confirmed reservation for the use of the diagnostic tool, which might incur supplemental delays, in addition to the waste of additional transportation. One of the incremental improvements in which the team should engage concerns the tuning of the quantities of tools and spare parts.
This is an area in which kanban excels as a means for low risk and evolutionary optimization. Mean lead times can be tracked against the volumes of tools and parts on hand to determine when optimal quantities have been reached. As conditions evolve, these quantities should be periodically rechecked, given that the flow of work tends to vary dynamically.
Summary
I have reviewed some of the particularities of field service as it relates to the management of the flow of work. I have then shown that kanban is fully applicable to the management of field service. However, field service has a set of special constraints that may require adaptations to kanban by a field service team. I have suggested various practices and tools, such as pre-commitments, mobile card boards, start time prediction plots, robotic process automation and working in pairs, that may be tested as means for addressing the issues of customer expectations, value stream waste and poor resource liquidity. It is up to each team to decide if any of these techniques address the real issues they have and how they may be adapted to meet the needs and maturity levels of those teams.
The article Kanban and field service by Robert S. Falkowitz, including all its contents, is licensed under a Creative Commons Attribution-NonCommercial-ShareAlike 4.0 International License.
Notes:
1 That being said, the customer’s expectations are likely to be framed by many types of bias, depending both on earlier experience with the provider as well as other types of previous experience.
2 See, for the case of pair programming, Alistair Cockburn and Laurie Williams,The Costs and Benefits of Pair Programming
3 The issue of how field service agents share knowledge has been discussed at length by Julian E. Orr in Talking about Machines: An Ethnography of a Modern Job (Ithaca, 1996).
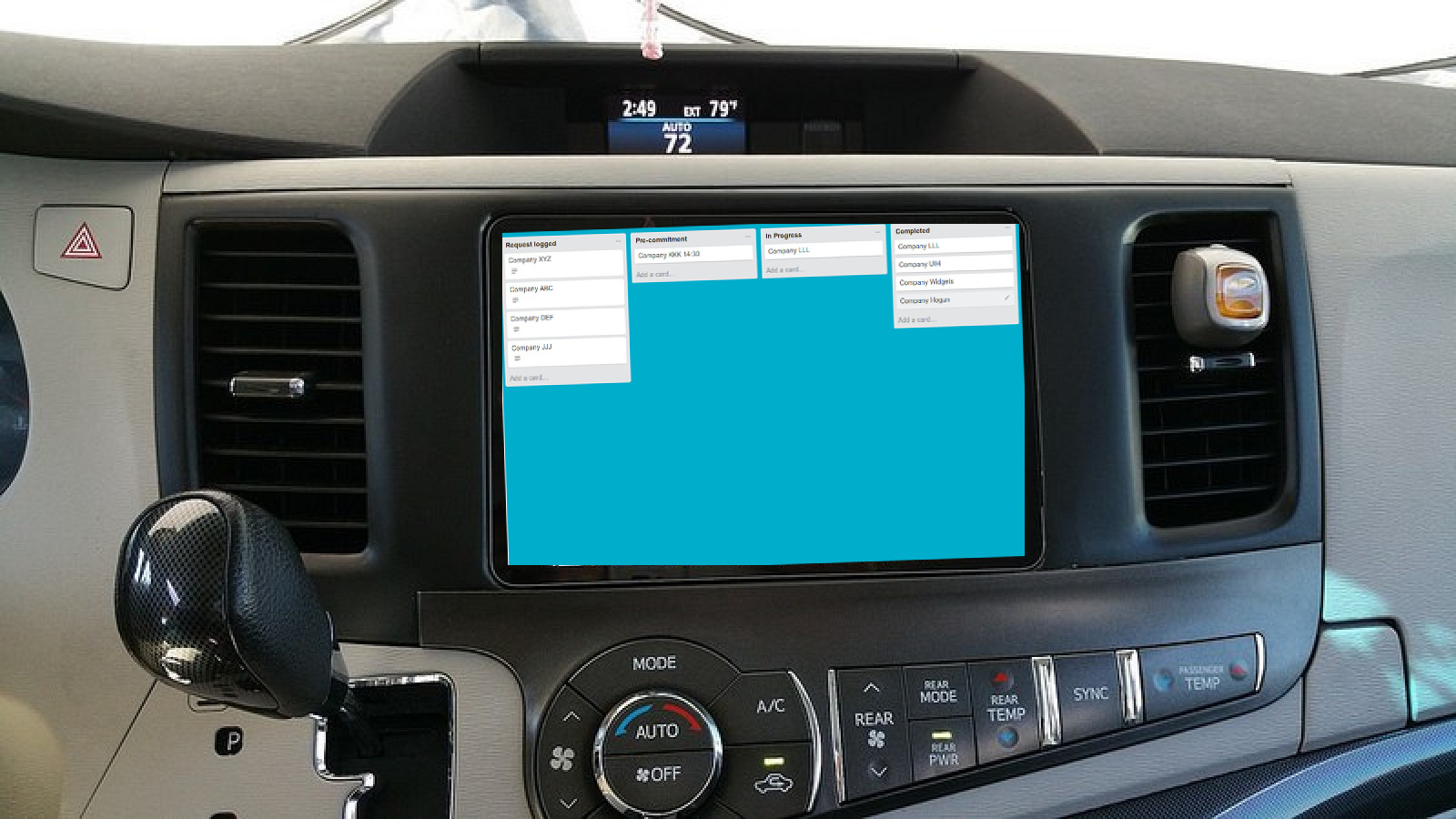

Leave a Reply