Is the data you record about managing services merely a by-product of other activities, just some administrative overhead? Do you want to benefit from artificial intelligence tools to improve your services? If so, you should consider establishing an AI data strategy in your organization.
The quality and the quantity of available data underpin the benefits of artificial intelligence. However, service providers often view the data concerning service delivery and service management only from an operational or even a tactical perspective. They view data as a by-product of service delivery and service management.
A strategic perspective on service management data specifically targets the breadth and quality of its production. Successful use of artificial intelligence to support these delivery and management activities depends directly on this data. Thus, the service provider would benefit from an AI data strategy. What do I mean by this?
To answer this question, I will first review the data issues that a service provider typically faces. As we will see, these issues undermine the usefulness of service management data in supporting artificial intelligence. I will then articulate various data strategies intending to address these issues. These strategies should provide a sound basis for using AI to support the delivery and management of services.
The Problem of Service Management Data
Consider how service providers often approach the management of the data concerning their management of services. They define a set of management disciplines, such as incident management, change management, problem management, etc. Each discipline handles a set of cases. Data records describe each case. One or more service management tools store these data records in their respective databases.
This approach leads to a variety of issues in data management:
- Focus on collecting data about non-value adding activities
- Multiple systems of record
- Focus on the volume of work rather than the quality of work
- Redundant data recording
- Devaluing service support roles
- Focus on inspection and correction rather than the right quality at the service act
Non-value adding activities
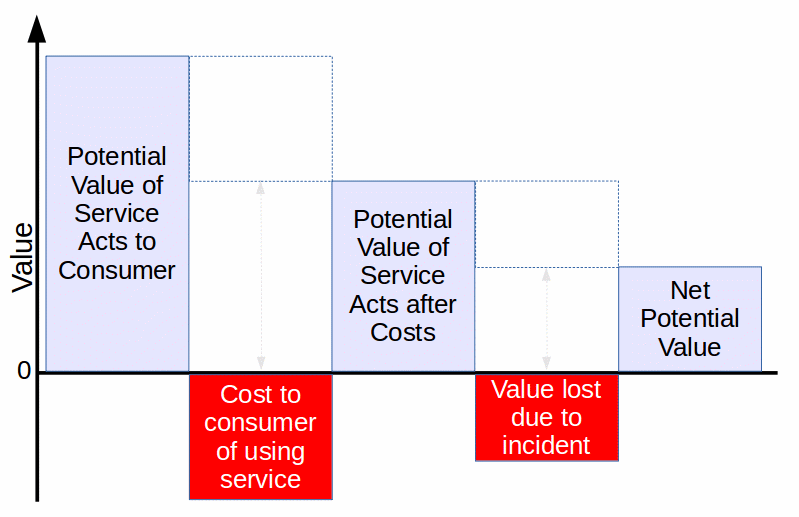
In almost all cases, these disciplines are non-value adding (as viewed from a lean perspective). In other words, they are concerned with preventing the destruction of value, rather than making positive contributions directly to the service consumers. Thus, handling a service incident does not increase the value of the disrupted service act; it only tries to restore normal operations in a timely way. Often, the incident destroys value in proportion to the length of time required to restore acceptable operations.
Handling a change concerns identifying the negative risks associated with the change and ensuring appropriate mitigation of those risks. Failure to identify and appropriately mitigate such risks increases the probability that performing that change will destroy value.

Looking at the other side of the coin, good handling of an incident nevertheless adds no value to the service acts that were disrupted. At best, today’s good incident handling can help tomorrow’s incident handling to resolve incidents with even less destruction of value.
Similarly, even though a service provider might perform a change under perfect control, that control would not make the change more valuable. Again, good change control might result in future change requests that better analyze and plan for risks, but it does not increase the value of the changes themselves.
Thus, the data collected from many service management disciplines (indeed, the disciplines on which most organizations focus!) cannot be the basis for improving how the service provider adds value.
Systems of record
Service providers generally create authoritative records of management activities based on the data collected about those cases. Those data allow service providers to compare their performance to benchmarks, to justify their investments in management resources and, one hopes, to guide improvement activities. The decisions made about handling cases determine which data are collected. Thus, those data reflect, at best, what was done, rather than what should have been done.
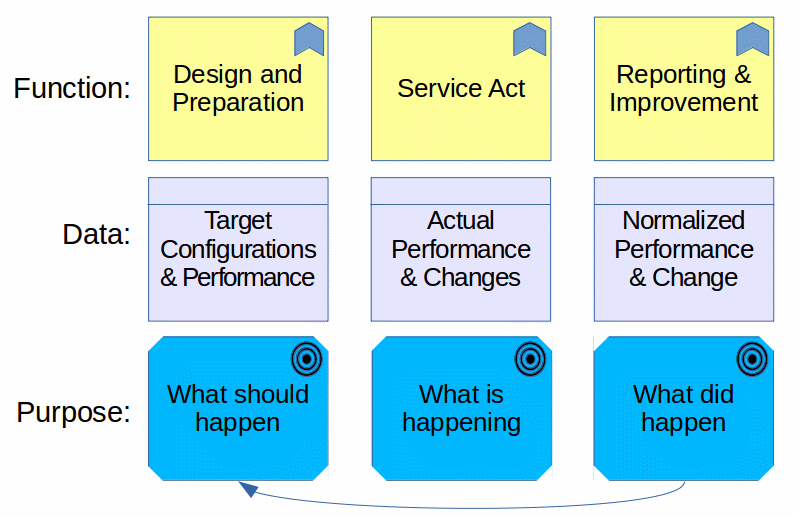
Post mortems, problem management, knowledge management and other improvement activities address the gap between what did happen and what should have happened. Often, what should have happened differs significantly from what had initially been planned. Initial planning never considers all possible eventualities. Completely unexpected events occur. Sometimes, a combination of anodyne events occurs in unpredictable ways. Also, the planning is sometimes incomplete or inaccurate. The result is that there is a split between the system of record that records what did happen and the system of improvement that attempts to shape what will happen, if there is a next time.
But which system is used to train artificial intelligence to support future service act performance? AI should be trained on the records of what did happen and what should have happened (according to a post mortem). All too often, the only the record of what was planned is preserved after the fact. The actors in the service act record in an unstructured way what did happen, if they record it at all. They record what should have happened, again, if recorded at all, in an abstract, generalized format. If the service management personnel record links between the records of what did happen and the record of what should have happened, such as the link between an incident and a problem or a change and an incident, those link structures have minimal semantic value.
Volume of work rather than value of work
Data collection during the handling of a case is problematic for various reasons. First and foremost is the pressure to work quickly. While this pressure might be most obvious for handling service incidents, it is present in all disciplines. This is because most managers tend to assess work in terms of the volume of cases handle per unit of time, rather than in terms of the value accrued to providers and consumers by the handling of those cases.
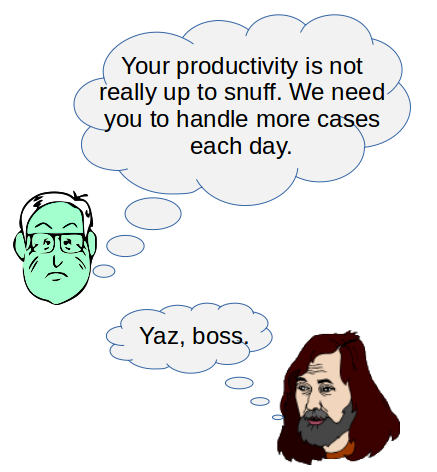

Service management tools and technology management tools

Another issue is the use of service management tools in parallel with technology management tools. Virtually all service system technical components, such as server platforms, network nodes or databases, have their own management tools. These tools are the primary interfaces between the components themselves and the specialized personnel managing those components. The architects of service management tools ask those specialists to record the same data that is available in those management tools. They impose a significant manual and redundant effort since there are hardly any automated interfaces between technology management tools and service management tools, excepting events or alerts. That effort is of little value to the specialists, who depend instead on their technology management tools for their perceptions of reality. Consequently, records tend to be incomplete and prone to contain errors of fact.
Low cost and high turnover
When considering the first line of support for service consumers (in organizations where this traditional structure exists), the issues are quite different. Too often, managers treat these support people as if they were fungible, with the result that they are thought to be easily replaceable and worthy of only very low salaries.
These two factors work against the need to develop experience in working with a service provider, learning about its culture, its services, its technologies and its consumers. Often, there is little investment in training and developing these people. If they gain enough experience to be effective, they quickly move on to other positions. In many organizations, communications between first and second lines of support (and, heaven forbid, the third line of support) are erratic at best. The first line sends work to the second line, which sends that work back because it is, in the view of the second line, incomplete, erroneous or misdirected. In spite of these issues, they hardly ever reserve enough time to transfer the knowledge that would be required to get the data recorded correctly the first time around.
A traditional command and control approach to managing data quality focuses, as we will see below, on inspection and data correction activities. But preventive improvement activities, too, are problematic because they are very poorly scalable. Since the individual experiences and knowledge of each support agent are likely to be very different, improvement needs to be tailored to the individual. Unwilling to invest in such expensive and extended development approaches, many organizations end up doing exactly the opposite of improvement. Instead of benefiting from the particular skills and knowledge of each agent, they seek to bring them all down to a least common denominator of behavior. Support becomes predictably humdrum and uninspiring.
Thus, the circumstances described above often impair the quality of the data recorded about the handled cases.
Inspection and Data Correction
Recognizing the issues described above, some organizations invest in data record inspection and correction as part of the closure activity in case handling. They justify this activity in various ways. It is a way of detecting quality issues in the handling of cases. Thus, it is a precursor to some type of improvement activity, a highly laudable purpose. Furthermore, by improving the quality of the data in the records, the reporting should be more accurate.
Often, someone other than the person who initially handled the case handles the inspection and correction of that data. This inspection activity may introduce additional bias to the data recorded. The initial data recorder already has certain biases concerning what data they deem worthy to record. The inspector does not have first-hand knowledge of the case. Thus, the corrected data reflects that inspector’s inevitably biased understanding of the documented issue. A discussion between the original data recorder and the inspector might mitigate the problem of bias, but how many organizations are willing to invest in what is essentially triple work? Furthermore, faulty memory tends to facilitate the introduction of biases, unless the inspection occurs very soon after the events.
Unfortunately, the inspection and correction approach comes too late to avoid the waste in the handling of the case. The situation is exactly as in the manufacture of goods. If you wait to the end of the production line to inspect the goods and throw away the defective ones, you do nothing about all the wasted effort that went into producing the defective goods, not to mention the cost of the wasted raw materials and the wasted intermediate inventory handling.
So it is with service delivery and service management. If you deliver the wrong service or if you handle a management activity incorrectly, you destroy value and damage your reputation. The moment of truth is during the service act. True, an improvement activity might help to avoid future cases of such damage. But that future improvement is of no use for the consumer who abandons the service provider, given the poor service in the past.
Operational and Tactical Uses of Data
In the discussion above, the service provider uses some data about service delivery and management in an operational way. The data are inputs that determine the nature of the service delivered. Service providers also use them to manage the flow of work. Thus, a traditional organization, with lines of support based on technology, may use case classification to manage the assignment of cases to one team or another.
Given the issues raised above, errors in the data may lead to delivering the wrong service or performing the service in an ineffective or inefficient way. Thus, service providers use these same data in a tactical way to detect errors, to prioritize issues and to support future improvement activities.
In spite of the issues I have raised, I hold these operational and tactical uses of service data to be extremely important and do not discount them. However, the service provider will make best use of artificial intelligence by applying it at the moments of truth during the service acts. This timely use of AI leads to greater efficiency, less waste and higher consumer satisfaction.
Artificial intelligence, such as is the state of the art today, depends heavily on the quality of the data that it models, the data that is the basis of its training. Rather than depending on a purely operational or tactical approach to these data, a service provider should have a strategic approach to acquiring and managing those data if it wishes to benefit fully from the advantages of artificial intelligence. Good data become sine qua non for good artificial intelligence, rather than being a luxury—great if you have it, but you can muddle through if not.
Recommended AI Data Strategy
Various disciplines, such as business intelligence, data science or business process automation, use data strategies that also support artificial intelligence. Although the service provider might already use some of these strategies, it should focus on their importance for the successful use of AI. Here are some of the key strategies:
- unifying all relevant data in a single data source
- basing decisions on detailed data, not aggregated data
- interfacing with technology management tools
- automating data capture
- using machine learning techniques to test captured data plausibility
- using a virtuous cycle of improvement
AI Data Strategy 1: Single Data Source
In the early day of service management practices (before ca. 2005), service providers managed their services using essentially two types of tools: the tools used to manage the technology and the tools used to manage the workflow. However, as service management gained traction as a distinct discipline, software designers developed more and more tools to support the goals of well managed services.
This flowering of technology also led to the storing of service-related data in many different tools. To allow machine learning to benefit from those data, the service provider should consolidate them within a single data source. Given the large volumes of data that a service provider might have available, the so-called “big data” tools and techniques might be required to achieve acceptable levels of performance. This is true both for training an AI and for using an AI in an operational context.
Before the use of AI and statistical learning techniques, service providers typically aggregated individual data sources into databases used for reporting and as systems of record (see Fig. 7). As we will see, aggregates cannot be the basis for AI use.


AI Data Strategy 2: Detailed, not Aggregated, Data
The complication of data sources described above led to the development of tools to synthesize the data and provide high-level analytical and reporting tools. Often, these reporting tools were based on aggregated data, rather than on the low-level data collected as part of service operations. They were the basis for the analysis of trends and reporting on service level objective compliance.
Service providers assume that aggregation permits insights into the data leading to more useful levels of information and better decisions. Furthermore, aggregated data is often the basis for reporting on systems of record, such as for financial statements. These data must be stable.
Although aggregation does provide certain analytical insights, it nonetheless introduces bias in describing data. This bias can destroy the potential for other types of insights. For example, if you report financial data aggregated according to a chart of accounts, you might have good visibility of the difference between the cost on sales and overhead costs, but you are not likely to have any visibility on the impact of cost reductions on sales, by customer segment.
In service management, it is common to aggregate data as counts of cases handled per reporting period. In problem management, you might report the number of open problems at the start and the close of January, as well as the count of problems resolved or canceled during that month. But this aggregation gives you no insight into how problems relate to each other or why one team might be successful in resolving many problems whereas another team seems to do nothing at all about problems.
In service delivery, it is common to report on the count of service acts, typically broken down by customer segmentation criteria. As important as that might be, these aggregates do not give a basis for assessing how and why the segmentation criteria might evolve over time.
AI Data Strategy 3: Automated Data Capture
One of the results of the segregation of service management tools from and technology management tools is that service management personnel must capture manually in service management tools much of the data already available in technology management tools. Each time a human agent manually copies data, that agent’s biases may unconsciously filter the data; may introduce errors in the data; and fail to complete data entry, or even fail to capture the data at all.
While some service management tools are provided with connectors that support the importing of data from other tools, these connectors tend to exist mostly for creating new records—especially incident records—based on events that monitoring tools detect. Tool architects do not generally design these connectors to query other systems for the purpose of completing a single field within a pre-existing record.
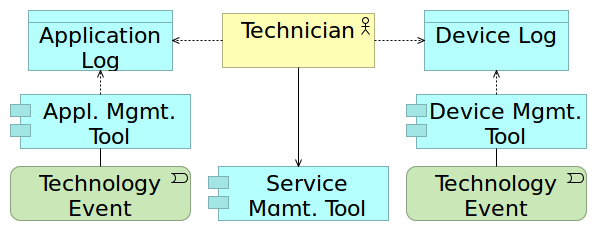

Service providers have difficulty quantifying the effort required to redundantly capture information manually. They can hardly measure the biases and resulting errors. As a result, they bring few arguments to develop such interfaces to automate data capture. Furthermore, if the personnel lack clarity on how those data will be used, they are poorly motivated to ensure the quality of such data.
Machine learning, however, is able to analyze very large amounts of data about complex interactions. It can find correlations that human agents could only find by applying lengthy data science data techniques. The incentive for automatically capturing large amounts of data about how service systems work is to provide the basis for machine learning analysis.
AI Data Strategy 4: Plausibility Checks
However much tool integration and automated data capture are in place, there will still be a large place for manual data capture. This is largely due to the unstructured descriptions of events and requirements coming from the various service stakeholders, especially the untrained, uninitiated service consumers.
Support personnel, too, are known to record text that is incomplete, erroneous or otherwise difficult to understand. Although it is a significant challenge, AI can help increase the plausibility of manually captured data, making it more usable for subsequent analysis.
The more a service system is complex and tightly coupled the more it is a challenge to test the plausibility of captured data. Take the example of analyzing the causes of a service incident or the risks of introducing a change to a service system. In a system with very few components, where the flow of operational steps is not continuous or is buffered between steps, analysts may easily identify which component might be the location of a failure. For example, if there is no power to a computer, it is pretty simple in the vast majority of cases to identify whether the fault is in a power switch, an internal power supply, a cable or somewhere farther upstream in the electrical supply. However, suppose there is power to a computer but it appears to be frozen, not reacting to any key presses or mouse movements. This problem could be the result of a combination of many simple events or statuses, each of which individually would not be a problem, would not cause a failure.
Now, suppose you want to capture a list of the components involved in a failure. In the power failure case, an analyst could identify with a high level of confidence which components are plausibly involved. It would be extremely unlikely (albeit not impossible), that an external loudspeaker be the cause of the problem. If a user, by inattention, were to select such a component from a list, it should be possible to signal to that user that that component is probably incorrectly entered.
In the case of a frozen computer, wherein many different components can interact in an unexpected way, it would be much more difficult to assess the plausibility of any single component as being involved in the problem.
So, we need to distinguish between assessments based on past events (is it plausible because it has happened before?) and assessments based on system analysis (is it plausible because it coheres with the structure and the dynamics of the service system?). Assessment based on past events hardly needs a machine learning solution to deliver a response, insofar as the actors have reliably recorded structured data about events. Assessment based on system analysis is difficult, insofar as system structures are often imperfectly documented and system behavior is imperfectly understood. However, analysts could exploit such algorithms such as Loopy Belief Propagation to calculate the probability of a node in a system being in a certain state, given what they know about the other nodes in that system.
How could a service provider use this information? Suppose an agent manually records data about an incident or a change. The management tool could assess the plausibility of those data display a plausibility signal, such as a color or a number on a plausibility scale (say, 1 to 10). A poorly plausible data set does not mean the recorded data are incorrect. Rather, it is a signal that the agent might think some more about the accuracy of the recorded data. A highly plausible data set does not mean the recorded data is accurate; it only means that within the limits of what is known about the service system, there is no particular reason to doubt the accuracy of the recorded data.
To return to the power loss example, suppose an agent describes a failure in a computer wherein no power is available. Suppose he or she records that the failure involves the use of a certain application because the user reports having been using that application when the failure occurred. The tool would then assess the plausibility of this information and display a warning sign. The warning does not mean that it is impossible for a locally running application to be the cause of a power outage. It only means that the relationship is so unlikely that it would be very inefficient to assign the incident to the administrator of that application. Of course, if the historical record starts to show a pattern of power outages while that same application is in use, then the plausibility would correspondingly increase. The interest of using an AI to make this assessment is that it would be less likely subject to human biases. I suppose that most support agents would never give a second thought to the seemingly ridiculous idea that a business application could cause a power outage.
AI Data Strategy 5: A Virtuous Cycle
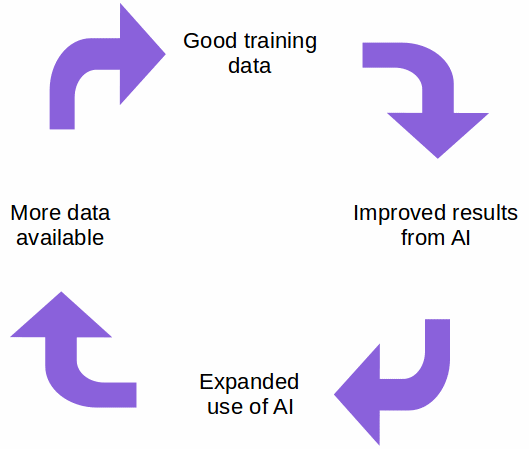
This virtuous cycle serves to influence cultural change in the organization. If the organization was AI-skeptical, it will tend to increase trust in AI-based solutions. If the organization viewed the capture of data needed for good classification as little more than administrative overhead, then the cycle will tend to convince actors of its value, both to the service consumers and to the service providers.
To get the virtuous cycle started, a service provider should trust to more than dumb luck and happenstance. If the data set used for initial training of the AI classification tool is incomplete and of poor quality, then the trained tool is likely to yield unsatisfactory results. Thus, it is important to encourage the strategy of high data quality as a precursor to implementing an AI tool.
Summary
Practices exacerbating the problem of capturing useful data have long plagued the management of services. These same practices will impact the potential benefits of artificial intelligence in helping to deliver and manage services. By applying the AI data strategy described here, service providers will develop a culture of effective and efficient data capture which, in turn, will support the beneficial use of AI.
The article AI Data Strategies for Service Management, by Robert S. Falkowitz, including all its contents, is licensed under a Creative Commons Attribution-NonCommercial-ShareAlike 4.0 International License.
Credits
All the diagrams are the work of the author


Leave a Reply